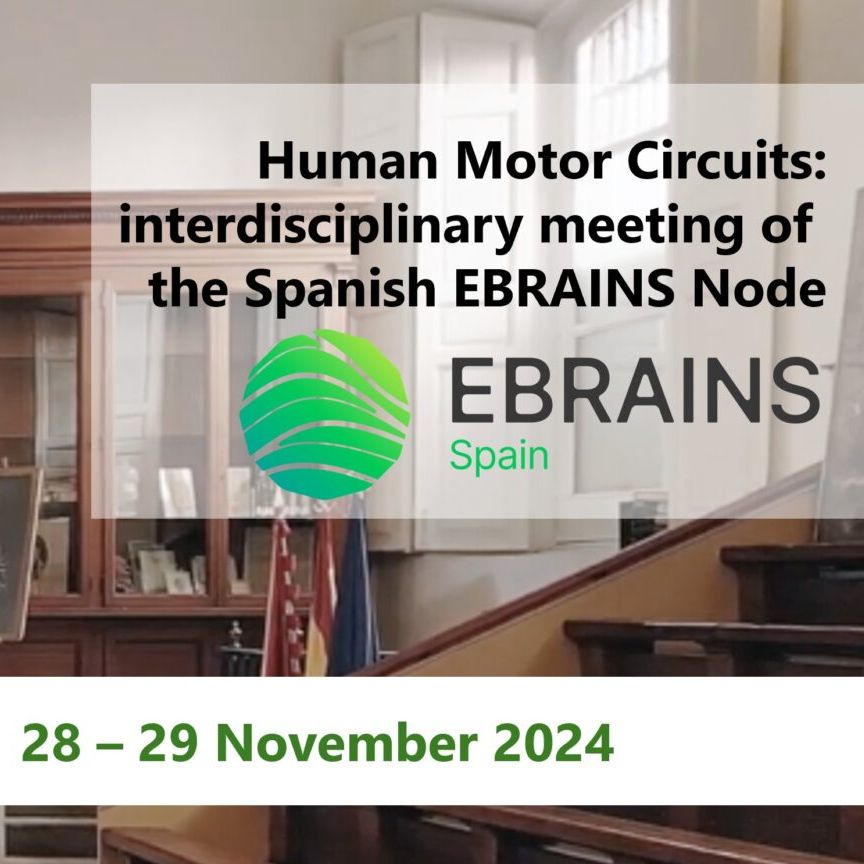
Training medical AI models with the Digital Brain Tumour Atlas on EBRAINS
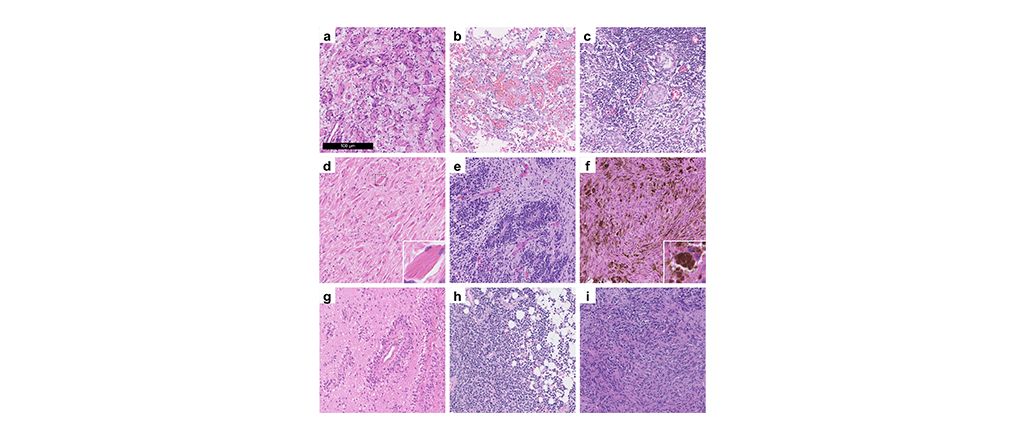
A large brain tumour database on the EBRAINS research infrastructure has been used in new deep-learning advances for cancer medicine. The results have been published in four articles in the renowned journal Nature Medicine. The Digital Brain Tumour Atlas used in these studies is an open resource contributed by the Medical University of Vienna to EBRAINS.
The World Health Organisation defines around 150 different types of brain tumours. This large diversity can make it difficult for clinicians to diagnose the precise type of a patient may have. Artificial intelligence methods have the potential to support diagnosis in the future. Training the algorithms and models requires a large number of accessible digital histopathological datasets. The Digital Brain Tumour Atlas (DBTA) on EBRAINS gives access to well-annotated data of 3,115 slides scans and 126 brain tumour types. The bank contains brain tumour data from cases – each with their own clinical annotations – recorded between 1995 and 2019. The 3.6 Terabyte of digital data are accessible through the EBRAINS Knowledge Graph.
Research teams in Australia and the US have now used the DBTA as one of the key reference datasets in high-profile studies about using AI for the rapid diagnosis of brain tumour types and on the mitigation of training data bias through general-purpose foundation models.
At the Australian National University, the scientists have used the EBRAINS data in the development of a new AI tool to more quickly and accurately classify brain tumours based on DNA methylation. Their model was trained and validated on large datasets of approximately 4,000 patients from across the U.S. and Europe. DNA methylation-based profiling is the current gold standard for identifying different kinds of brain tumours, but requires time-intensive tests that are not always feasible. The model provides a way to predict DNA methylation and subsequently classify brain tumours into 10 major subtypes with an accuracy of 95%. It offers the potential to aid and accelerate the clinical experts' diagnosis as a complementary tool.
At Brigham Mass University Hospital, researchers compared different specialised AI models trained on the Cancer Genome Atlas and the DBTA on EBRAINS. The scientists highlighted differences in their performance for different demographics, pointing to biases that need to be mitigated for making AI-based diagnostics more balanced and fair. Previously the same researchers had used the DBTA on EBRAINS also to benchmark brain-tumour subtyping for two universal foundation models of pathology trained on over 100 000 slide scans of different tissues. The team was able to show that these foundation models can reduce the differences in performance that were observed in the specialised AI models and enhance accuracy. The research highlights the potential and need for training medical AI models on a larger scale with more extensive and diverse data.
"The rapid pace of AI adoption in medicine highlights the importance of creating large, well-curated scientific and clinical datasets in Europe, which are needed for AI foundation models", comments EBRAINS Joint-CEO Katrin Amunts. “EBRAINS supports such initiatives with world class FAIR data services and supercomputing infrastructure.”
The EBRAINS data-, compute- and atlas services aim to play a key role for AI applications in neurology and provide high-quality data in a privacy-compliant ecosystem. EBRAINS also makes high-resolution histological and multi-modal data accessible to improve personalised brain models.
"We provide the digital environment and expertise to bring data, models and computing power together including the entire support chain, i.e. data curation support, the provision of sophisticated metadata standards, GDPR-compliant data protection solutions and access to computing power," says Katrin Amunts. With federated systems, we can facilitate the processing of large and diverse training data across Europe, which promotes generalisability and performance."
Related news items:
24 Feb 2022 Brain tumor data now available on EBRAINS
https://www.ebrains.eu/news-and-events/brain-tumor-data-now-available-on-ebrains
08 December 2022 Pre- and post-surgery brain tumour data available on EBRAINS
https://www.ebrains.eu/news-and-events/pre-and-post-surgery-brain-tumor-data-available-on-ebrains
Original publications:
Hoang, DT., Shulman, E.D., Turakulov, R. et al. Prediction of DNA methylation-based tumor types from histopathology in central nervous system tumours with deep learning. Nat Med (17 May 2024). https://doi.org/10.1038/s41591-024-02995-8
Vaidya, A., Chen, R.J., Williamson, D.F.K. et al. Demographic bias in misdiagnosis by computational pathology models. Nat Med 30, 1174–1190 (19 April 2024). https://doi.org/10.1038/s41591-024-02885-z
Lu, M.Y., Chen, B., Williamson, D.F.K. et al. A visual-language foundation model for computational pathology. Nat Med 30, 863–874 (2024). https://doi.org/10.1038/s41591-024-02856-4
Chen, R.J., Ding, T., Lu, M.Y. et al. Towards a general-purpose foundation model for computational pathology. Nat Med 30, 850–862 (2024). https://doi.org/10.1038/s41591-024-02857-3
News & events
All news & events- 13 Nov 2024
Brain Innovation Days 2024
Event - News10 Oct 2024